For the past half-decade, the field of People Analytics has undergone significant growth and has been adopted by a number of organizations. The organizations that have developed advanced skills in analytics are witnessing positive business outcomes and better employee experiences.
Teradata Vantage contributed to the growth of People Analytics by empowering Teradata’s platform with NewSQL, Machine Learning and Graph Engine which has enabled users to build an end-to-end analytical pipeline on a single platform.
One of the leading telecom companies of South Asia wanted to leverage the power of People Analytics to predict which of their key employees were likely to churn and to identify those employees who has the potential to play a pivotal role in organizational success. The company was also going through a digital transformation and merger with another local telecom company.
Based on the data sources available from employees’ profiles, different behaviors and traits of employees were identified to establish a baseline foundation. All the data sources were staged in Teradata. From LinkedIn and LinkedIn Learning profiles, things like prior experience, total experience, education, skills, trainings and interests were extracted through a web scrapper. Data exploration and profiling was done with Teradata DataFrames using Jupyter Notebook. The next step was the segmentation of employees in which scoring was done based on their performance, engagement activities and contribution toward the organization which also included training and development of individuals. The top 10% were marked as High Profile (HiPo) employees, the next 60% were marked as Medium, and rest as Low. Following the segmentation phase, an ADS was built and featured selection was carried out in Vantage. Multiple machine learning algorithms were implemented and, based on the results, the best model was selected to identify employees who were likely to churn in upcoming quarter and those employees who had the potential to become HiPo in the coming year.
Insights were built based on the predictive results.One of the most interesting insights showed that the biggest chunk of predicted churn is within the first 2 years of employment (23%) and the second biggest predicted churn is between 10 -12 years of employment. It was suggested that policies should be devised to retain these employees. Suggestions included offering different loans, automotive financing and other incentives which can bind employees to a company for a longer period of time.
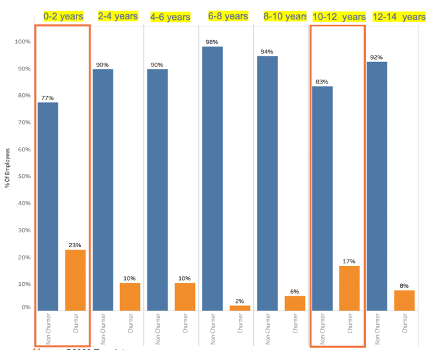
Another insight brought to light was that a particular department was predicted to have the highest churn (28%). For this department, the average predicted churn was an estimated 7 years as opposed to the general organizational trend of a 1-2-year employee lifecycle.
.png)
Results showed that predicted churners at the most junior grade -- Level 1 -- in this department had gotten less percentage of recent incremental salary raises as compared to the rest of the employees in the same job grade. The churn at this level was predicted to be 9% and the tenure of these predicted churners was less than non-churners by 3.74 years. The churn at level 2 was 10%; employees were predicted to stay longer in Level 2 before churning. The age of predicted churning employees was lower than average as well. For level 3, employees who were younger than the average age were predicted to churn. Their tenure was less and they were given less incremental raises, which resulted in a 14% predicted churn for level 3. There was not any significant difference in the variables’ distribution for the level 4 grade, but still the highest churn predicted was for this grade was 30%.
.png)
Based on the age of the employee at the time of joining, the biggest chunk of people who have the potential to become a top performer joined between 25 to 28 years of age. A significant decrease was visible after this age bracket. This showed that employees who joined the company at earlier ages were more career oriented and put in an effort to mark their footprint within the organization. This also indicated that employees with a certain amount of prior experience had the potential to perform better.
Employees joining from telecom and banking sectors showed potential to become HiPo; yet employees from a computer software industry background didn’t show potential to become top performers within our analysis.
Most of the employees did join the company with a certain number of years of experience; and a major chunk of employees had 4 years or less of experience before joining. Employees with 10 to 14 years of prior experience were most likely to be Top Performers if percentages are taken into consideration.
.png)
The results achieved by this model were promising. The predictive trend analysis depicted the actual circumstances of the organization related to employee engagement and attrition. The Human Resource department devised policies and strategies to control the employee attrition by using the analytical model, which in turn brought up the retention policies.
On the other hand, this solution also made the management accountable as they had to justify prominent churn figures under certain managers. The outcome of the model also highlighted short comings in the employee evaluation process.
Collectively, the model increased human resource management awareness within the organization and allowed the management to focus on areas that could prove promising in employee retention and satisfaction.
Faraz is a Data Science Consultant with over 11 years of experience in successfully delivering analytics and information systems solutions / projects to leading telecommunications, retail, FMCG and banks across Pakistani, MEA and North American markets. Faraz has led the successful inception, design and execution of advanced analytics projects covering different industry use cases including market basket analysis, people analytics, performance prediction modelling, customer journey and path analytics. Faraz has also held technical lead roles on several data science projects in a multi-platform ecosystem environment. He is currently focusing on building Teradata Assets over Vantage.
View all posts by Faraz Shahid