Invariably, one of the most frequently asked questions I hear from customers and prospects with respect to analytics is, “Do you enable real-time analytics or batch analytics?” I’m not sure what people really mean when they ask this question—and I don’t think they understand either. Are they talking about how often a model is scored? When it actually drives a decision? How relevant the decision making data should be? Too often people believe that the algorithm updates itself or “learns” in real time after it’s deployed. (It doesn’t, but we can tackle that topic in a future discussion).
Ask people to give an example of a “real-time analytics” solution and many will point to one of the most useful analytically-driven solutions today—the search engine. A tremendous amount of R&D, data, and analytics drive your everyday search engines. We’ve all used them. They are extremely helpful and certainly provide answers in as close to real time as we can practically hope to experience.
Nevertheless, to understand what’s happening in real time, let’s take a very high-level view of how a search engine works and see if it can help us make a distinction between real-time analytics and batch analytics. My apologies in advance to search engine vendors out there for my over simplification.
Five Step Process for Search Engines
First, search engines must map the internet and collect data. They do this constantly and use the data as reference points to relate search criteria with internet objects (pages, context, images, etc.). The amount of data collected in this endeavor is almost unfathomable because, according to
Internet Live Stats, there are nearly 2 billion websites. Search engines look to map all of these pages and their objects as well as relationships between related pages and objects—using processes that have literally run (and continue to run) for decades. Like most solutions, these can be considered a means by which data is captured and made available for some future use. This is an ongoing exercise. We can refer to this as
general data management.
Second, analytics are applied to show the strength of various online object relationships, identify where people are trying to artificially improve their relationships, and apply other types of analytics to make sense of the data that is captured and will be used to map to search criteria. New data is put through algorithms to update the level of confidence, but the algorithms are relatively static until a new model is deployed. The value of the output is a function of the initial model development (and training) and the vast amount of data used to process the analytics. Because these outputs support the automated feedback generated by the search engine, we refer to this step as analytical automation.
Third, search engine providers use their own analytical “secret sauce” to provide predefined ranking scores based on provided criteria and use other analytics to augment these scores. This recommendation score can be augmented in the later step, but it’s a process-intensive workload. Trying to rank every single possible search criteria with every possible web object is not something that can be run in “real time.” Instead, recommendations are preprocessed or values used to make a final ranking recommendation are determined beforehand. We refer to this as the recommendations.
Fourth, in a step called personalization, many search engines use personal information that denotes where the person doing the search is located, content of previous searches (using things like cookies), predefined preferences, and vendor investment (to push vendors higher up the search results list). Any or all of these factors can impact what someone sees to better suit their individual preference and search relevance. This information is defined as a series of rules by the search engine to individualize predetermined ranking recommendations.
The final step is decisioning, one where the person types in search criteria in real time and gets back results. In this step, an analytic technique called, “Natural Language Processing” or NLP, is deployed to understand the context of the request and reach out to the knowledge base to pull back the required results. At this stage, recommendations, rules, and other decisioning criteria provide a personalized view of the search results and capture the results of the link that’s picked (which is used as part of the ranking recommendation process).
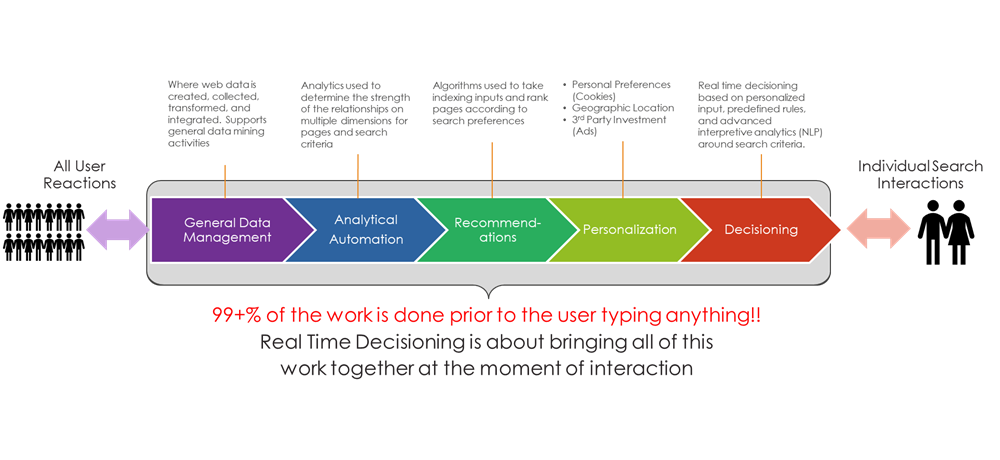
Right Time, Not Real Time
In our simplified example of a search engine, we see two very important things:
- Almost all the work to provide the result was done long before anyone typed in search criteria.
- Analytics are applied at multiple points in the process. Which means that when most people talk about real-time analytics, they’re really talking about real-time decisioning. In this case, much of what is learned about the person making the search request cannot happen quickly enough to run all of the analytics necessary to make a decision. Please see the following example below:
I created a framework titled
Automating Intelligence, to evaluate how to develop an analytical solution by applying analytics to support a business process. The
Automating Intelligence framework is applicable if you’re looking to drive a real-time decision or perhaps something not quite at the moment of interaction. How do you decide? Well, it depends on the business process and the cost/benefit analysis to see what level of latency is necessary. Even then, the vast majority of work that provides context to your analytical decision is done well before the decision needs to be made.
Stephen Brobst stated it well when he pointed out to an audience at the recent
Teradata Analytics Universe, “It isn’t about
real time, it’s about the
right time.”
In conclusion, the next time someone asks you, “How do I use real-time analytics to solve my problem?” make sure you ask them to clarify, “What problem are you looking to solve?”
Tom Casey is Executive Account Director for Teradata. He has nearly 25 years of experience working with, designing solutions around, and helping global customers make analytics actionable. As a data analyst, Tom has successfully implemented the use of statistics to better segment and target customers in support of major corporate programs. He’s a featured speaker at conferences, author of several papers, and has a solid track record delivering enterprise-scale analytical solutions.
View all posts by Tom Casey