A closer look at COVID-19 data
The dire statistics on the COVID-19 epidemic had me go on a tailspin of worry, anxiety, and frenzied reaction. I was buying masks, disinfecting the hell out of everything that I would touch and generally being the stay at home wallflower that did not quite come naturally to me. All this in direct response to headlines and statistics that essentially promised a life filled in purgatory if I did not take the right precautions now.
To be clear, the COVID-19 pandemic is indeed dangerous. It ought to be taken seriously; and it is likely to cause immense personal, economic, and societal damage were it not to be treated with the abundant care that it deserves. But, after marinating in so much data, a part of me wonders if we are all reacting to the immediacy of the data and the rabid conclusions we could draw out of it:
Should we not be casting a more critical eye on many of the data-driven conclusions presented to us?
Let me give a few examples first to illustrate the genesis of my concern. The
table below gives the top 10 nations ordered by COVID-19 diagnoses as of September 18, 2020.
If one concluded that the USA is perhaps the worst affected simply by looking at the total figures, one could be immediately forgiven. However, it must be pointed out that affliction numbers are proportional concomitants of overall populations. Simply put, greater populations imply greater infection numbers (and greater infection numbers are an outcome of more testing too as you will see later). Looking at data like these in isolation tends to also inflate concerns without much logical underpinning. On the face of it, it looks like the impact of COVID-19 in Argentina is about a tenth of the USA’s as is the case with Spain. But, is that really true?
Let’s look at normalized figures after we compute the total number of cases per 1 Million individuals in a country.
By looking at cases per 1M in population, we see a few significant changes: For example, India’s meteoric drop from being 2
nd overall to the 10
th position or a relatively small Peru claiming the top spot when one computes a density metric. Spain and Argentina have now moved to the middle of the pack.
Now, ask yourself what the implication of this is when you mull over your plans for how to deal with rising numbers of the pandemic across different regions.
Indeed, let’s take it one step further, and ask ourselves if the case numbers on a “per million” basis are truly reflective of the underlying actuals or if they are muddied by some other moderating variable such as, say, testing.
Ah, testing – that much vaunted holy grail that is yet to be made available in vast numbers and with ease across most of the world. Let’s look at the numbers below:
.png)
A-ha (I had to get a nod in to my favorite Swedish band not named ABBA), the numbers above make me scratch my head. The USA has the highest number of cases in total as well as on a per capita basis while also having conducted the greatest number of tests among any nation in the world.
Could it just be that the there is a greater number of infections detected in the USA simply because more tests have been done?
Conversely, are nations with smaller totals – be it as a grand total or on a per million basis – showing those numbers because not enough testing has been done or is it because their populations have not been infected as much? Take the case of India which has one of the lower infection rates despite being the 2
nd most populous nation in the world. However, India has also one of the lowest tested population on a per capita basis. Now, our basic analytics intelligence quotient makes us dig deeper to understand if and how diagnosis outcomes are indeed connected.
Are infection rates lower because not enough tests have been administered or is it because of policies that promote social distancing, reduced travel, sealed borders, in addition to rigorous testing or some combination thereof? How much does one counteract the effects of the other? What are the interaction effects?
In fact, if you look at the table below that shows diagnoses per each Million of a tested population the initial conclusions could open themselves to drastic modifications:
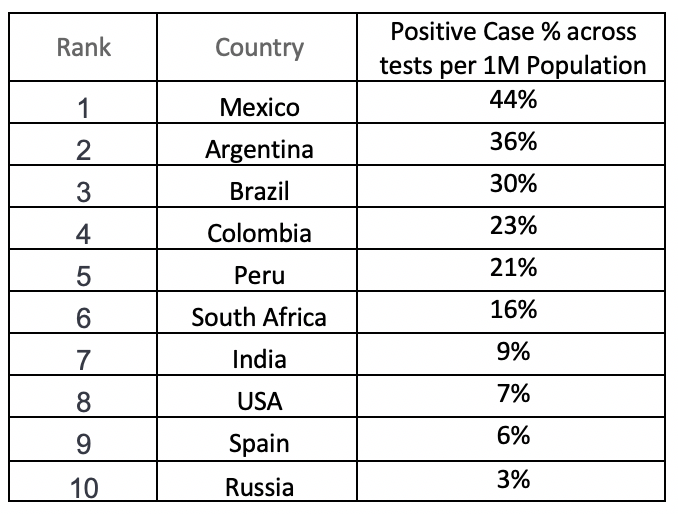
Mexico shows a whopping 44% infection to testing ratio for every 1 Million of its population. But, there is the issue of causality here: Are we seeing more cases because we are testing more people randomly? Or are we only testing the individuals mostly from high risk population cohorts? Or is it a mix of the two and to what degree and how do we separate out the effects? These questions and their implications are largely ignored as well in the media. It is a bit of a red herring to conclude that this number will be the same for every subsequent 1 Million as we do not know a lot about the people tested or how the testing is even done. However, we can look at a relatively large proportion of tested individuals showing a positive result as one possible indication of the likelihood of accelerated transmission across the population, and hence, a cause for concern.
But the larger point is, as we add more data, more nuances keep getting added that were absent when the initial conclusions based on one global metric was considered. And, while that is a good thing to note, in this simple example, it is also sobering given that most attention-grabbing headlines seem to be making conclusions based simply on that one global metric (too many news sources to enumerate). To repeat, none of this is to make the point that we should not take COVID-19 seriously. Far from it. Even one death or debilitation is one too many. However, it is dangerous as well to make decisions without fully looking at the context of more than one data point or source. This has the effect of misallocating precious resources that could be used for optimal treatments, rehabilitation policies, preventive care, economic relief and more. The implications of bad analyses have real world consequences for us all. And I am not even going to address here, the unseemly case of data being willfully suppressed by vested interests when accounting for national statistics.
5 best practices towards better analytical reasoning
So, the obvious next questions are, how do we not fall into this analysis trap and what steps could we take to be a bit smarter in how we look at data. There are many things we should do, and this is not meant to be an exhaustive list. But here are a few things that have worked for me. Some of these, no doubt, are more common sensical than anything else but I have lost track of the numbers of times these have been more honored in the breach than I thought possible:
-
Understand the question that the analysis is answering and see if you can come up with logical follow ups
In the COVID-19 case we are trying to understand the true prevalence of positive tests, the severity of community spread, and the likely impact to a population for a finite future. Let’s take the non-COVID-19 example of customer churn. It is one thing to simply find out how much churn has occurred in the last year. It is another thing entirely to ask who churned and when; if the churn was different across customer segments; if there are unique segment level differences in common variables that could account for these varying churn metrics; if there are seasonal differences, and so on. Asking a composite set of questions where each subsequent question is tightly related to the previous conclusions makes a big difference to the kinds and qualities of decisions taken.
-
Understand for yourself how your measures are defined
Can we all be sure that a test for the virus is administered exactly the same way in each of the geographies that we are looking at, particularly at the early stages of this hitherto unseen pandemic? If tests are widely different then how do we interpret the numbers per million in each population? What do the metrics even mean when they come out of different measurements? As a non-COVID-19 example, let us take the case of Net Promoter Score (NPS). NPS scores are the difference between the positives and the negative scores from a survey. However, a negative, in some surveys, is defined as anything 6 or lower on a scale of 1-10. Other NPS measurements have different calculations. So, how can we correctly interpret a specific NPS score if we do not know the underlying measurement logic?
-
Include other contextual data
Often times data sets are constrained not just in volumes but also in depth and richness. This is not all that bad if you accept that the insights delivered from the analysis are limited to the data collected. But we can do better. For example, in the context of COVID-19 testing, we could include another data point such as when region wide lockdowns occurred. Doing so, now enables us to connect infection outcomes to another variable that now widens our ability to make clearer policy prescriptions. Or, conversely, adding more data could eliminate certain pre-conceived notions of causality that were readily assumed to be true. Either way, we get a much tighter analysis. To go beyond healthcare, on the NPS side, again we can add geospatial data, for example, to layer on top of the NPS scores to see if there are geographic variations and then inquire further as to the nature of such differences.
-
Know your data sources
In one of my recently read works of fiction, “Cloud Atlas” by David Mitchell, I was struck by a brilliant line “He who pays the historian always calls the tune”. Besides, heartily recommending this book to all you book lovers, this line is particularly relevant to how data are sourced. Despite our best efforts, most of us have been in situations where data are often corrupted by certain biases that creep into how they are collected, recorded, and enumerated. For example, a recent survey in Santa Clara County, California, where I live, showed the number of infected to be much higher than official numbers. The sample was obtained via an advertisement on social network for testing volunteers. This ignored the fact that a person at higher risk is more likely to sign up for the free test to get informed of his/her situation. Similarly, on the non-COVID side election polling is always fraught with issues when sampling bias is ignored. Imagine taking an opinion poll of only those people in your social circle and whose political stances are closely aligned with yours. Of course, the result of that poll will likely be different from the underlying actuals. Other types of biases that creep into the data include confirmation bias, model over/under fitting, and the non-inclusion of confounding variables.
-
Pick the right analytics for the job
In this day and age where there are so many analytic tools that have proliferated and the rise of a resume-based culture when picking tools to do analysis, the temptation to go fancy and rogue is all too consuming. Not every problem needs to be tackled with an AI algorithm. While a large bobcat (not the wildcat but the large machine used in construction projects) and a standard handheld shovel can upturn your garden’s soil, the former could be an overkill when we are looking at a 50 square foot area. However, the bobcat is powerful and novel and the temptation to use it and endure the expense of using it (particularly when someone else is paying for it) is too much to resist. But a rational reflection would ensure that we eschew the bobcat in favor of the simple shovel to do the job as it was intended. This is a metaphor for how we choose tools for analytics. In over 90% of the cases, a simpler set of algorithmic choices can do the trick. Making this choice has the crucial benefit of making your analysis accessible to deeper outside scrutiny, wider consumption, and a greater likelihood of being operationalized correctly.
Calls to action
Without a doubt, I have scratched only the shallow depths of best practices for analytics. There are many more that I can think of. I’d like to also learn from you and would appreciate your additions to this list. But what are some things you could do today, even in this context of the COVID-19 situation, that could make you look at data with a bit more purpose.
- Look at all data that you can get and start looking at their provenance? Who created it, how were the features extracted, who sponsored the study, why is the data including the variables that it is including?
- What is the data purporting to show? Are there alternative explanations for the conclusions given in the analysis?
- Are there other data sources that needs to be added that could shed more light on the current situation?
- Could alternative decisions be made were more data be analyzed?
- Could the insights be used to convince decisionmakers to change course?
While I want to wish away this baleful pandemic that has been overstaying its welcome, I know wishes alone don’t count for much. I wish for more of us to look at data critically, even with a degree of cynicism, and keep asking all the hard questions that a lot of you have been asking. All this could lead us to insights that are more reflective of our current exigencies and, more crucially, being able to operationalize those insights in a manner that delivers on a maximal good. Stay safe and healthy.
Sri Raghavan is a Senior Global Product Marketing Manager at Teradata and is in the big data area with responsibility for the AsterAnalytics solution and all ecosystem partner integrations with Aster. Sri has more than 20 years of experience in advanced analytics and has had various senior data science and analytics roles in Investment Banking, Finance, Healthcare and Pharmaceutical, Government, and Application Performance Management (APM) practices. He has two Master’s degrees in Quantitative Economics and International Relations respectively from Temple University, PA and completed his Doctoral coursework in Business from the University of Wisconsin-Madison. Sri is passionate about reading fiction and playing music and often likes to infuse his professional work with references to classic rock lyrics, AC/DC excluded.
View all posts by Sri Raghavan