The unprecedented outbreak of the COVID-19 pandemic has generated a lot of interest in the analytics community and a flurry of data collection and analytics work has come out in the last couple of months. We, at Teradata GDCs, also envisaged a data-centric and analytics-enabled early warning system solution for governments and other administrative organizations. Such an early warning system would depend on integrating a set of diverse data sources, such as census data, health data, mobility data and crowdsourcing data, and then running analytics on these cross-industry integrated datasets. Since Teradata is known for its leading data warehousing technology that allows for such large-scale integration, and its Vantage analytics stack that naturally leverages this underlying parallelism, we are best positioned to provide such a solution in a scalable and efficient way.
Solution Overview
The heart of our proposed solution (Figure 1) relies on two complimentary risk analytics models that profile individuals in the population and overall localities defined by geographic and administrative boundaries according to their likelihoods of being affected by COVID-19 respectively.
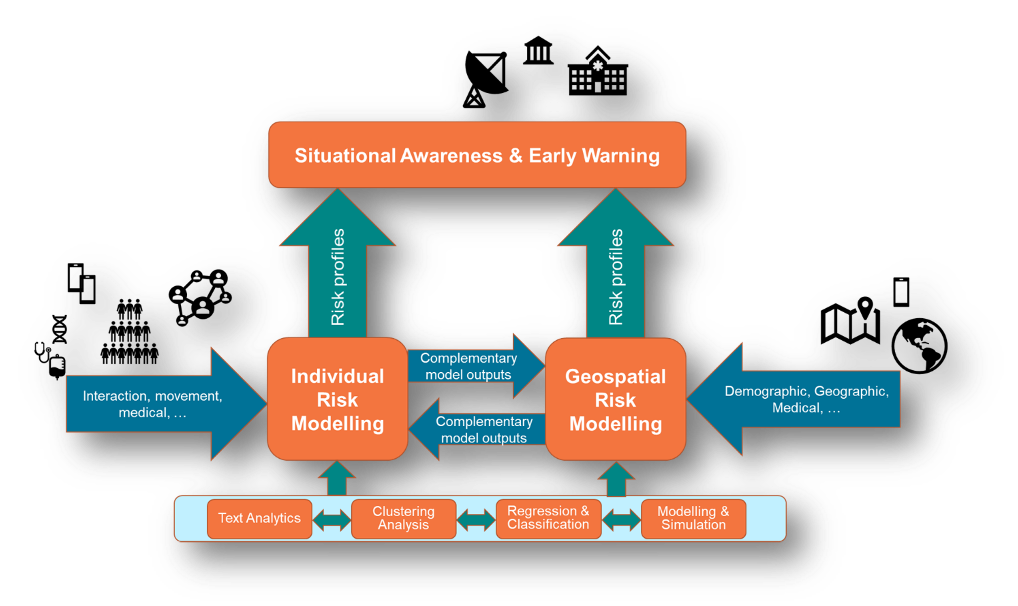
Figure 1: A risk-profiling based early warning system to control the spread of COVID-19
Enabling Analytics
The two risk models are enabled by a set of analytics that include:
Text analytics which focus on identifying prevailing and emerging indicators underpinning COVID-19 spread using intelligence gathered from news, technical reports, research publications and social media.
Figure 2: Identification of emerging COVID-19 symptoms and indicators through frequent term analysis of text data collected daily from different sources.
Figure 3: Word co-occurrence analysis to identify emerging COVID-19 indicators and symptoms.
Figure 4: Topic modelling to identify emerging themes related to COVID-19.
Figure 5: Sentiment analysis of Twitter data to identify emerging risks according to geographic locations.
Profiling analysis which focuses on characterizing different types and stages of COVID-19 cases and identifying the vulnerable segments using demographic and health data, as well as characterizing population mobility using data from telcos and social media.
Figure 6: A Individual and locality profiling dashboard using WHO and JH data sources.
Figure 7: Geographic profiling based on ratio of positive cases to total tests and case fatality rates using WHO and JH data sources.
Classification, regression and other machine learning-based analytics which focus on predicting COVID-19 contraction in individuals and its spread through geographies.
Figure 8: Predictions for COVID-19 deaths using ML algorithms using data from Kaggle and Philippines Government.
Modelling and simulation that focuses on analyzing COVID-19 spread dynamics, carrying out what-if analysis and evaluating options for effective and efficient resource planning in order to curtail the spread of COVID-19.
Figure 9: A mobility model based on probability distributions of people's movement to different areas based on their age.
Figure 10: A visual representation of a risk scoring model based on age of an individual and probability of their coming in contact with other individuals
Figure 11: A visual interface to simulate people movement in a hypothetical grid area. The colors of the cells represent different locales such as residential and education areas. The width of the white circles represent population densities.
In addition to feeding into risk engines, these analytic modules complement each other to improve their performances and accuracies. For example, the profiling analysis module provides useful input for building realistic simulation models for the disease spread. Similarly, the insights gained from text analytics could enrich the profiling and machine learning modules through additional features and indicators for model inputs.
Risk Models
The risk models take input from enabling analytics modules and generate risk scores both at an individual as well as geographic levels. The risk scores to individuals are assigned based on their likelihood of contracting COVID-19, transmitting to other individuals and recovering from the infection.
The geographic risk scores are assigned based on the overall mobility levels in and across jurisdictions and the proportion and magnitude of infectious population in the area.
Both models allow us to develop an early warning and situational awareness system which authorities can use to warn individuals based on their movements through mobile phones or other communication channels, as well as trial and test curtailing strategies for COVID-19.
Figure 13:The risk scores of different population zones in the simulated hypothetical grid area based on people's movement, vulnerability indices and several other factors.
Prototype
A team of data scientists from three GDCs (Pakistan, India and Philippines) have developed an early prototype demonstrating the above concepts using data available in the public domain, which includes COVID-19-related data from WHO, John Hopkins Institute, Kaggle, Twitter and several national web portals. Some sample visualizations coming out of different streams of analytics work are shown above in Figures 2 to 13. 100% of the data, including the raw data pulled from public domain, the refined analytical data sets and the data used for visualizations, is staged in Transcend – Teradata’s internal platform to test and refine products. We achieve this by focusing on providing a technical analytic ecosystem that is recognized as best in-class and positioned as a customer. A Covalent interface is also being developed that will be used to host the front end of the early warning system integrating all analytic outputs under a single source. The application of this product is well and beyond the COVID-19 risk alone and can be used to monitor any future emerging risks.
A special thanks to the following people for their contribution to this solution and article:
- Fitzroy Dy, Data Scientist, GDC Philippines, who worked on the profiling;
- Madhuri Patil, Data Scientist, GDC India, who worked on the text analytics;
- Muhammad Jawad Khokhar, Senior Data Scientist, GDC Pakistan, and Kailash Talreja, Data Scientist, GDC India, who worked on the modelling and simulation part of the solution.
Kamran is a seasoned data scientist with a PhD in machine learning and AI and more than 15 years of experience working in different industries. He is currently a principal data scientist with Teradata GDC, Pakistan. Prior to that he has worked in several senior data science roles, including as an independent consultant, with the Australian Government and with other academic and research organizations in Australia. Kamran’s expertise includes a range of machine learning, optimization and simulation technologies including deep learning, evolutionary computing, multi-agent systems and reinforcement learning. He is currently leading several data science projects including the one for predicting and containing COVID-19 spread.
View all posts by Kamran Shafi